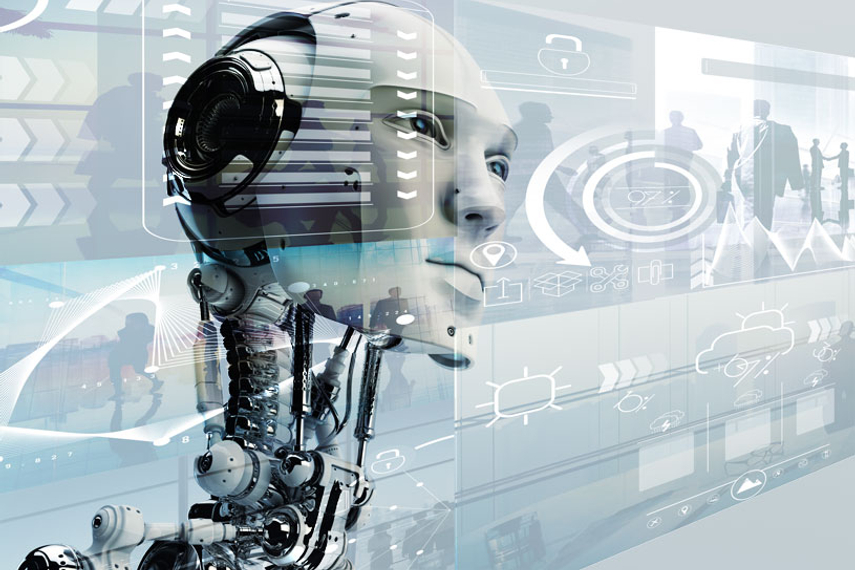
Modern companies want to know everything about their connected clients: who they are, what they feel, who they work with, work out with, sleep with, what they want to buy, when, why, from whom, at what price and so forth.
Online companies within travel, hotel, music and dating have to use advanced analytics of client behaviour to survive in competitive businesses. They keep tweaking their systems — such as online client analytics and the client offering at the center of the online business model — in short project cycles. Truly digital companies like Spotify and Tinder regularly analyse hundreds of millions of events to understand clients and remain one step ahead of them, on an individual level.
There is no shortage of companies promising to deliver ‘actionable intelligence’ by means of advanced analytics of ‘big data’ — which has yet to deliver on its hype. The hype cycle for new technologies can be explained by visions running ahead of necessary conditions for success.
In the dotcom years, ventures failed because reality, or rather, connectivity, had yet to catch up with the vision of the internet as a place for shopping.
Big data has so far failed to deliver on its hype. However, the confluence of three trends is now setting the stage for a transformative shift: the first trend is the exponential growth in data, which is doubling every 24 months; the second is the proliferation of smartphones and the behaviour of hyper-connected users (the average user now checks their phone 150 times a day); and the third, and the most significant, is the emergence of computers that are learning to read.
|
While the first two trends are already well-known, the importance of the third trend is still not widely understood. This, I would say, has been the missing component for success when it comes to big data analytics.
Data growth and smartphone usage are of no use if computers don’t ‘understand’ most of the data. Until now, computers have only been good at dealing with structured data, which may explain why big data has yet to deliver on its hype. The bulk, or 80 per cent, of all data is, however, unstructured and consists mainly of language data.
We are now seeing the emergence of machines that learn and understand this kind of streaming data in a meaningful way and on an ‘internet scale’. It is a giant leap in the evolution of computers and guarantees that big data analytics will over-deliver on its hype.
The usefulness of big data — let’s define it here as ‘data so large or complex as to create a challenge when applying traditional methods’ — will exceed expectations and even overhyped big data activities will impact businesses across sectors.
One such activity is sentiment analysis. The latest generation of self-learning and concept-based technologies has huge potential, as opposed to traditional methods that use training data and wordlists.
It makes sense out of large streams of language data and is used to understand how populations, their subsets and micro segments feel about anything in real time. It is used for predictive analytics, which is about patterns and statistics. Weather forecasting is a fair analogy: accuracy has improved enormously with the help of more and better data and with the power of computers.
The new tools have proven impressive. Predicting outcomes in parliamentary elections, the distribution of viewer votes in televised contests and even with short-term predictions for financial risk assets.
The latest tools for alias matching, profiling, segmentation and predictive analytics are able to handle vast data streams from different sources. These machines are capable of learning by reading and understanding the meaning and patterns in a better and more useful way.
Traditional industries have started to look around for ideas. Banks, for example, have successfully increased profitability with the help of advanced analytics. Unfortunately, these initiatives tend to become the management’s secret pet project and thus lessons are not shared and expensive mistakes are repeated — holding back the industry.
However, if more widespread use of better analytics comes into play, companies will be able to predict and act on consumer trends much faster. As a consequence, trend cycles become shorter, faster and more segmented — down to the ‘segment of one’. Unknown brands can rise faster and established brands can decline more quickly.
Data analytics is changing the dynamics and the competitive landscape across most industries. Client ownership is becoming more volatile. Businesses will need to be more analytical and agile in order to stay one step ahead of their connected clients, to secure loyalty from both customers and clients.
Similar to earlier technological shifts, this will be an opportunity for your business if you choose to embrace it and leverage its potential.
Lars Hamberg is a big data expert with over 20 years of experience in the financial services industry.