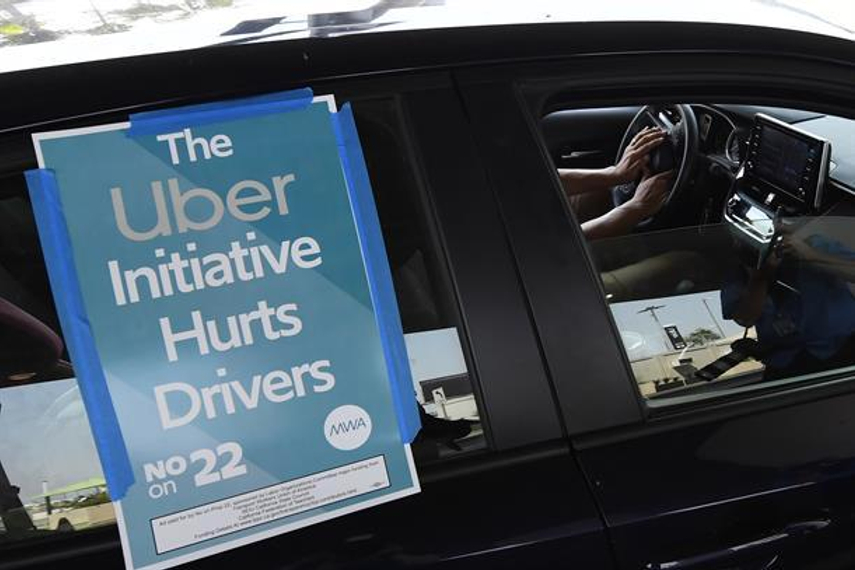
Please sign in or register
Existing users sign in here
Having trouble signing in?
Contact Customer Support at
[email protected]
or call+852 3175 1913
We need to work with the algorithms, not for them.
Contact Customer Support at
[email protected]
or call+852 3175 1913
Top news, insights and analysis every weekday
Sign up for Campaign Bulletins
The grades are in for Campaign Asia's 22nd annual evaluation of APAC agency networks. Subscribe to read our detailed analyses.
Havas's post-split independence will be on trial. Can its ‘Converged’ strategy deliver business growth, and can its leaders address the internal diversity issues?
PHD clinches Bunnings’ $50 million media account from Initiative in a fiercely contested multi-agency pitch.
Gartner's 2025 CMO Spend Survey finds average marketing budgets are at 7.7% of company revenue for a second consecutive year. However, half of CMOs reported budgets of 6% or less.