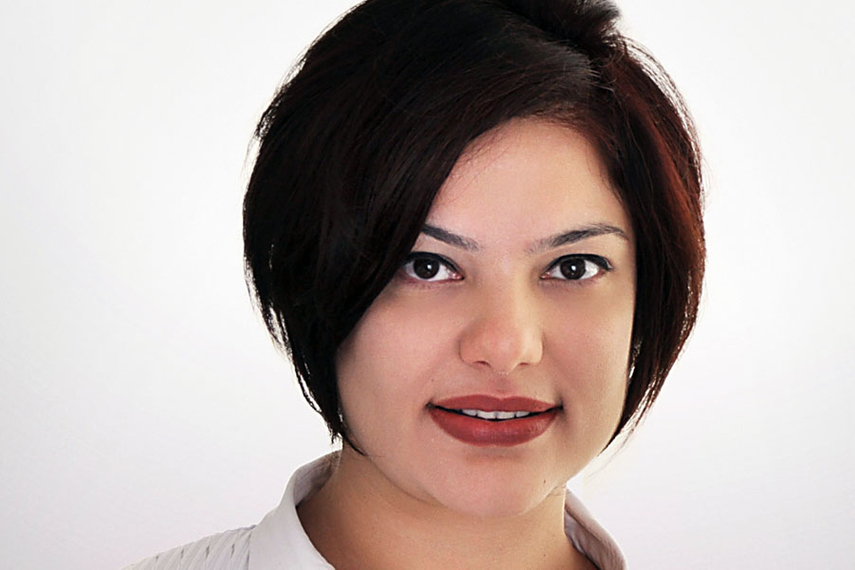
Today, every organization—especially in the banking and financial sector—is sitting on huge amounts of customer data. With the immense popularity of data science and machine learning, the business world is just beginning to tame this data deluge to its advantage. When exploited correctly, this data can prove to be extremely useful.
Data versus actionable intelligence
Traditionally, banking and financial institutions have been terrific at gathering tons of data about their customers. At any given point in time, they have access to hundreds of variables about their customers, such as demographics, family details, employment details, financial details, and property details. In addition, they also have number of related "environment" variables like source of lead, legal factors, market conditions, and so on. This is a huge amount of information about any individual customer or institution. But is it enough while deciding whether to lend or not?
Historical data has shown that this mountain of information is not sufficient. Because it cannot help the financial institution "know" its customers deeply. For instance, it does not tell the bank what the customer's interests are.
Why does predictive analytics matter?
Financial lending is a risky business. It is difficult to ensure that the bank is approving loans to the right businesses and individuals. Also, it is difficult to measure credit risk and predict the likelihood of a default.
Predictive analytics involves the use of available data, statistical algorithms, and machine learning, and allows banking and financial institutions to extract relevant information from the noise, and use it to predict behavior patterns and trends. And predictive analytics can provide a company with a leg up over other players, because it not only helps to make better and faster decisions, but also strongly improves marketing efforts (by identifying cross-sell and upsell opportunities), helps retain valuable customers, and prevents future fraud.
Building successful predictive lending models
With smart devices and mobile technology invading every aspect of our lives, a very important input for building a strong predictive lending model would be location data. This data can tie together the multiple data sources, correlate them with data science models and deliver risk parameters for the borrower.
For instance, location data—when overlayed with search data or spend data—can help institutions delve much deeper into their potential customers' profiles. The place or location where an individual lives, studies, or works can help derive significant conclusions about their lifestyle (or affluence level.)
Similarly, knowing their travel patterns is another meaningful way of extracting lifestyle information. Do they travel often? Is their travel mostly within a city or a country or around the globe?
These are but a few data points for banking and financial institutions to understand their consumers' behavior and interests to build a context around their need for borrowing. This information can be crucial in building accurate predictive models to identify and analyze potential borrowers, and reach out to them at the right moment and the best places to retarget them to build brand awareness and recall.
An example
Let’s say a bank wants to proactively identify and target potential home buyers in a specified region to boost revenue from home loans.
By using a location-aware data platform, and combining its first party data, the bank can get insights like where its target consumer resides, whether the resident is renting or owns the property, whether the resident is looking to upgrade the home and whether the resident is interested in investing in a property. These consumer-specific insights, can help the banking and financial institutions build a predictive statistical model to identify potential borrowers. By analyzing the lifestyle and behavior patterns of their consumers, the bank can pinpoint and target the right individuals and start the conversations with this audience even before they started looking out.
Last words
Location data has emerged as a strong variable in building and strengthening high-end predictive lending models. And it is more important than ever for these banking and financial institutions to leverage this new data for credit-risk analysis and credit-risk management, which are fundamental to these institutions that offer loans to individual, retail, and small-business customers. Banking and financial institutions, among others, need to wake up to the abundant opportunities available to strengthen their customer base and also manage their existing relationships with customers efficiently, before their competitor makes the move and steals their base.
Aditi Kohli is general manager for SEA and HK at Near