.jpg&h=570&w=855&q=100&v=20170226&c=1)
There is a resurgence of Media Mix Modeling (MMM) in the digital marketing landscape due to privacy changes like the loss of cookies and mobile IDs.
The top three advertising platforms, Meta, Google, and Amazon, have invested in MMM, a statistical tool for measuring advertising effectiveness, including acknowledging the impact of competitor channels. MMM uses aggregate data to evaluate a wider range of channels across the digital and traditional spaces, and across seasonality, promotions and other such variances.
This approach marks a departure from typical practices, as major ad platforms seldom use tools like MMM, which evaluate the performance of various advertising mediums. Traditionally, brands conduct MMM using statistical models to assess sales and media expenditure data.
However, recent privacy regulations have significantly limited measurement capabilities, prompting these large platforms to adopt any viable measurement tool to demonstrate attribution.
Meta initiated this trend three years ago with the launch of Robyn. This open-source MMM tool does not depend on cookies or personal data, coinciding with Apple's App Tracking Transparency framework, which hindered platforms from tracking conversions, severely impacting Facebook.
Amazon finds its first-party retail data advantageous for MMM analysis, while Google is exploring the development of an MMM tool to assist brands in assessing advertising campaign performance across channels.
Campaign explores the renewed interest in MMM and how major platforms ensure their involvement in MMM analyses.
Is history repeating itself?
MMM existed for decades before real-time bidding (RTB) became prominent in adtech and the Internet. One can think of MMM as solving a puzzle with missing or damaged pieces.
Today, major players like Google, Facebook, Amazon, TikTok, Apple, and various retail media networks like GrabAds and Carousell Media Group have a solid incentive to prove that their advertising channels drive sales and help marketers reach the right audiences.
They can also provide valuable insights that help marketers better understand their customers.
By demonstrating these capabilities, these platforms aim to attract more advertising dollars and, in some cases, lucrative cloud workloads for data, storage, computing, etc, explains Niraj Nagpal, an ad tech and martech consultant.
In the long run, this strengthens their position as must-haves for any diverse media strategy and IT provider.
"Anytime you have data in different silos, be it a media channel, or cloud environment, the puzzle gets harder and harder to complete," Nagpal tells Campaign.
"At best, marketers will have to accept imperfection and bias that the data they do get (typically hashed or aggregated to remove personally identifiable information to be privacy-compliant) from these environments comes with expectations for an increase of media spend, and is often gated to a select few of advertisers that have the proper budgets, data science teams, and technical environments in the cloud or clean room of choice to ingest data for MMM use cases."
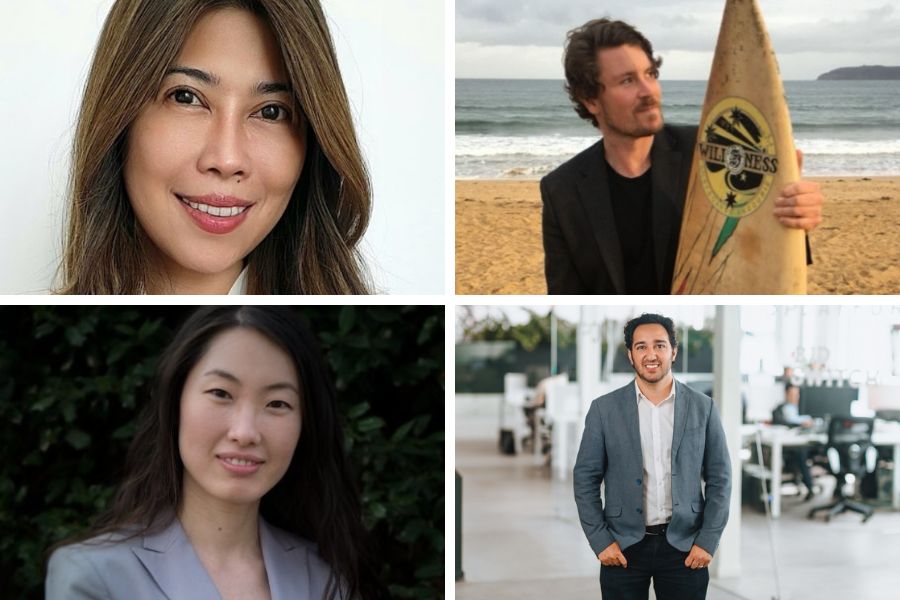
Nagpals recommends that marketers acknowledge the existence of these issues and work towards a unified data strategy, whether through a data lake or warehouse, and work with partners that provide privacy-compliant interoperability to enhance their already robust first-party data.
He warns that this process is not straightforward, and achieving an ideal state can be challenging.
Nevertheless, the initial step toward achieving a genuinely unbiased MMM is taking control of where marketers' data sits and partnering with agencies or teams capable of extracting insights from imperfect data sets.
"The advancements in machine learning and generative AI across the board will make it easier to gain insights from these complex data sets, where media teams without AI capabilities can focus on the narrative and value of data and not the specific inputs of data transformation, cleaning, consent management, etc," explains Nagpal.
MMM methodology and accuracy
In an environment where user-level tracking is increasingly restricted, marketers need to ensure the accuracy of MMM measurement.
According to Di Wu, the vice president of data science at Jellyfish, ensuring the accuracy of MMM can involve several straightforward approaches.
One way is to combine MMM with methods like experiments, which help measure the incremental impact of media. This data can either constrain MMM output or serve as a prior for Bayesian-style MMM modelling (an interpretation of the concept of probability in which in lieu of frequency, probability is interpreted based on knowledge or belief), enhancing accuracy by incorporating real-world results.
However, Wu warns that simply aiming for the best statistical scores like MAPE or R2 does not guarantee the most accurate MMM.
She explains an effective MMM should align with business objectives and not recommend drastic shifts in media investment that might surprise stakeholders.
"Business insights can steer the model results or create business-focused metrics with statistical measures, helping select the model that makes the most business sense. Accuracy is balanced with real-world business considerations," Wu explains to Campaign.
Larraine Criss, the chief operating officer at Preciso, points out the key is to focus on quality data collection, noting that automation can help improve accuracy and speed.
She says brands can ensure accuracy by focusing on their collected, aggregated data quality and incorporating appropriate attribution systems in their analysis.
"MMM methodology, such as multi-linear regression analysis, is usually used. In contrast, data sources from the brand's marketing activities, such as ad spend and ROAS, are collected to measure the effectiveness of their MMM modelling," Criss tells Campaign.
Beyond the ethical issues associated with user-level tracking, MMM's two limitations are scale, notes Liam Brennan, managing director at The Responsible Marketing Agency.
He explains the volume of data analysed that represents audiences and a tendency to over-index the short term, for example, an activity that drives sales versus brand building.
"MMM is by no means perfect, but with the right, accurate and scaled data, it can better represent both the short and long-term effects of advertising activity and, therefore, help marketers make faster and wiser decisions," says Brennan.
Platform-specific data sources and transparency
As data collection is a significant challenge when building MMM, platforms like Google, Meta and Amazon offer data feeds designed for MMM purposes.
Wu notes these feeds have some advantages. For example, platforms can provide more detailed data, including daily information at the local market (DMA) level. They also offer historical data spanning several years, enabling comprehensive analysis.
Google for instance, provides data going back three years, which marketers can download in a single go.
Accessing this data is relatively straightforward. Meta, for example, offers a user interface (UI) and API options for downloading data, making it convenient for MMM practitioners. It can be downloaded by marketers manually or scheduled for automatic retrieval.
In addition, the data feeds maintain consistent naming conventions, making it easier to differentiate between different types of campaigns and ads. For example, Google's data includes bumper and non-skippable ads for YouTube.
"However, it is important to note that these MMM data feeds are limited to the respective platform's data. Collecting data from other media platforms, including offline media, is necessary to build a comprehensive MMM model," Wu says.
"Additionally, data related to sales, competitive insights, events, and promotions should be gathered separately to ensure a holistic view of marketing performance."
Criss does not want to speculate about what is happening behind the scenes at Meta, Google and Amazon. She says providing measurement tools seems to be a focus for them.
However, it raises whether marketers let these platforms grade their homework.
Criss stresses the need for marketers to ensure accuracy when working on any data project and think carefully about the overarching plan and the actionable intelligence sought.
In this way, marketers can set themselves up for success with robust governance, avoiding shortcuts which are often expensive in the long run.
"It is essential to remember that MMM is designed as a data-driven solution to help marketers improve media performance and quantify the impact of marketing and brand investments," explains Criss.
"Thereby helping to determine the incremental effect associated with marketing activities and enabling the ability to use these findings to answer strategic marketing questions. Thinking strategically is critical. In-housing, where appropriate, may have clear benefits."
Criss adds: "MMM is not always the answer. What these models can do is form a crucial part of your overall measurement framework, but they should never be the only tool used to inform marketing effectiveness."
Role of AI and machine learning
MMM is seeing a renaissance in the context of AI and machine learning (ML), with technological advances potentially providing enormous benefits.
For example, ML automates crucial data tasks like cleaning, formatting, and merging data from diverse sources, leading to significant time savings and enhanced accuracy.
Advanced algorithms in ML can also uncover intricate patterns and interrelations within data, such as the influence of weather on sales or how seasonality affects advertising effectiveness, offering insights that might be elusive to human analysis.
As traditional MMM methods require time-consuming construction and testing of multiple models, ML can streamline this by swiftly evaluating different variable combinations, allowing for faster model development and easier updating with new data, significantly benefiting marketing strategy optimisation.
However, AI alone cannot solve every problem, while the most technologically sophisticated models will likely come with a high cost attached, warns Criss.
For example, to ensure compliance with privacy regulations, such as General Data Protection Regulation (GDPR), measures must be in place to protect user data while conducting MMM.
"The tide is turning increasingly towards privacy, and one of the critical reasons MMM analysis is growing in popularity so rapidly is that it does not require user-level data," explains Criss.
"MMM is a marketing measurement tool that does not rely on individual-level data, but offers the ability to enable marketers to make reliable data-driven decisions with up-to-date information and simulations."
Wu explains Jellyfish uses various media measurement methods, each with its purpose, to see a well-rounded view of our marketing efforts.
The agency uses multi-touch attribution (MTA) for ongoing optimisations of digital media to determine how to attribute credit to different parts of its marketing, which helps the agency make informed adjustments to its advertising strategies.
In addition to MTA, Jellyfish conducts incrementality experiments and conversion lift studies to answer specific questions and find opportunities for increased revenue. The agency tests these with significant platforms like Meta and Google and uses a geo-experiment method to measure incremental effects.
"The MMM tool guides our high-level budget and channel decisions. MMM differs from MTA as it does not rely on individual user data. Instead, it is based on aggregated data and considers various channels, strategies, and custom segments," says Wu.
"We also incorporate external factors like competitor promotions, Covid-19, inflation, and industry trends that may impact our business. A media planning tool is also used to forecast results based on available budgets and the model for future quarters."
Wu continues: "We use measurement methods to ensure accuracy and objectivity in our marketing analysis. These tools are not meant to replace each other but work together to understand our marketing performance comprehensively."
Future trends and developments
The future of MMM looks promising as it evolves to meet new challenges and expectations, as marketers are seeking more from MMM despite its long-established methodology.
Marketers will want more detailed insights, such as audience-specific, campaign-specific, and tactic-specific analysis, to understand the impact of each component in the marketing mix.
MMM models can also be sensitive to small changes in input or setup, so marketers will have to strike the right balance between art and science, ensuring the model aligns with existing business expectations while providing meaningful insights.
As MMM prioritises lower funnel tactics, it is challenging to quantify the impact of brand-building activities like CTV advertising and organic content, so marketers must find ways to evaluate these brand-focused efforts.
In addition, as previously mentioned, MMM models are typically built on historical data, which can become outdated as marketing strategies evolve rapidly. Marketers must quickly adapt to recent campaign launches to stay relevant and valuable to the business.
"Building MMM models traditionally takes time and manual effort, making it less agile in a fast-paced digital marketing landscape," explains Wu.
"We anticipate the future MMM to bridge the gaps in granularity, speed, privacy, and brand impact measurement while providing valuable and actionable insights in a rapidly changing digital marketing landscape."
Criss notes future trends will likely focus on reinventions of MMM thanks to AI and machine learning. After all, she says, while the discipline dates back to the late 1960s, today's automated data flows make it far easier to gather data from many marketing channels and information sources.
With historical spending and conversion metrics data, building a model with relatively low investment is possible.
"Typically, options include a media agency's solution, an independent provider, or in-housing. While there may be some efficiencies of scale for more prominent brands, the discipline is eminently possible without huge budgets," explains Criss.
"As with anything, research, a considered, strategic approach and due diligence are needed; in advertising measurement, the industry has evolved significantly and offers fresh solutions from smaller businesses - often thanks to technological advances."
Criss adds: "Meanwhile, advertisers are increasingly investing in data science teams. The trend is also similar to what we have observed in disruptive programmatic ad buying, which is helping to open the doors to premium advertising for all advertisers, democratising access to high-quality, qualified traffic at wholesale prices for merchants without the deep pockets of household names."
In addition, Criss says there is a need for industry-standard metrics or benchmarks for MMM to ensure consistency and comparability across brands and platforms.
"The Marketing Science Institute (MSI) has begun an initiative to set industry standards for marketing mix modelling, with John Lynch, its executive director, describing the landscape as a modern-day 'Wild West'," concludes Criss.